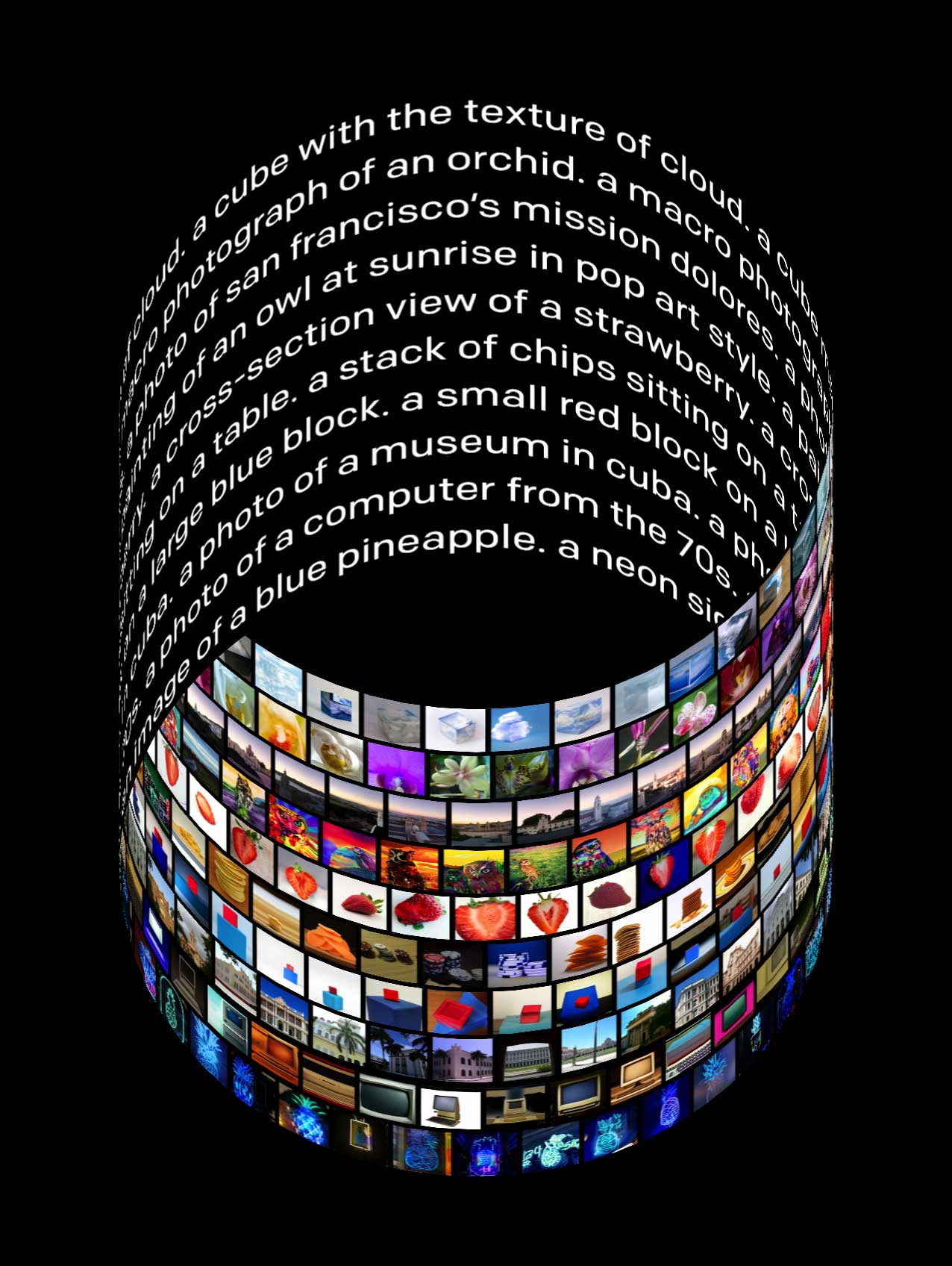
Are different genders fairly represented when generating images using OpenAI's Dall-E-2 text-to-image platform?
Since the invention of the multi-layer perceptron network, there has never been a time when AI has been more prevalent or visible in our society than the present day. Machine learning is used for almost everything; healthcare, business analytics, correctional systems, scientific exploration, and even in seemingly mild and light hearted applications that have achieved widespread commercial and critical success. One of these applications is the image generating model known colloquially as ‘DALL-E 2’, a software that receives prompts in the form of natural language and outputs its own perception of what the user wants to see. At first glance this seems completely fangless and friendly. However, as other investigations have demonstrated, this is clearly and concisely not the case.
Occupation | N | % Non-Male |
Financial/Investment Analysts | 387 | 40.2 |
Janitors/Building Cleaners | 2183 | 40.2 |
Lawyers | 1141 | 38.5 |
Cooks | 2012 | 38.4 |
Dentists | 140 | 36.6 |
Bartenders | 457 | 59 |
Biological Scientists | 110 | 57.9 |
Secondary School Teachers | 1000 | 58.7 |
Pharmacists | 375 | 59.6 |
Trainers/Fitness Instructors | 234 | 62.9 |
Occupation | Observed Proportion | P-value |
Financial/Investment Analysts | 1 | < 1e-6 |
Janitors/Building Cleaners | 1 | < 1e-6 |
Lawyers | 1 | < 1e-6 |
Cooks | 1 | < 1e-6 |
Dentists | 0.87 | < 1e-6 |
Bartenders | 1 | < 1e-6 |
Biological Scientists | 0.67 | < 1e-6 |
Secondary School Teachers | 0.42 | 0.022592 |
Pharmacists | 0.60 | 0.002961 |
Trainers/Fitness Instructors | 0.57 | 0.04494 |
Through our work, our group has proven the existence of significant occupational gender bias in OpenAI’s DALL-E 2 model. Several prompts did not even generate a majority female-presenting response, even when the data obtained from the BLS indicated that the profession was female dominated. While the impacts of such extreme bias may not be immediately apparent, as the model begins to see more widespread use across various applications, the impacts will likely grow more severe and visible. As such, OpenAI should move to address the bias present in their algorithms as soon as possible, before they see widespread commercial use.